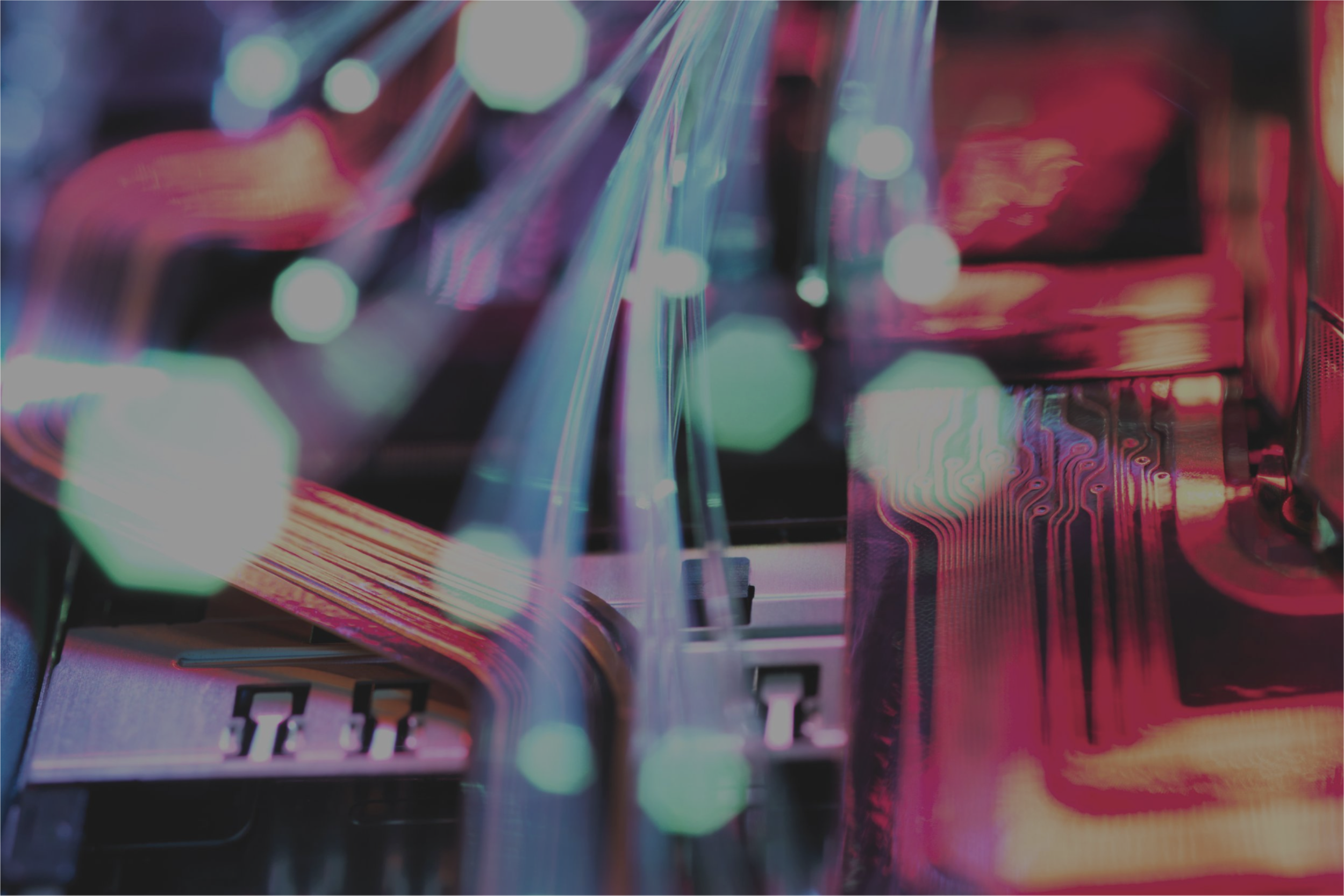
The FT Tech for Growth Forum is supported by
Foreword
Jonathan Derbyshire, Tech for Growth Forum Editor, Financial Times
This latest report from the Financial Times Tech for Growth Forum addresses a fundamental question facing companies today: how do we foster innovation in order to drive growth?
Businesses already know that the returns which flow from using advanced technologies such as artificial intelligence are considerable. The key for leaders is understanding how to unlock that potential by optimising their investment in research and development.
This report draws on expert testimony to offer companies a strategy that will take them to the cutting edge of technology and innovation.
AI and the R&D revolution
By Lucy Colback
In a world of fast-changing consumer preferences and increasing choice, companies that want to stay ahead must work continuously to ensure that their goods and services satisfy the customer. In 2023 McKinsey said that big industries, including automotive, telecoms and consumer products, anticipate that a third of sales, worth $30tn over five years, will come from new products.
Advancement is key and the level of fresh funds flowing into research and development is considerable. According to the latest UK statistics, £71bn was spent on R&D in 2022, of which £50bn came from the business sector. In the US the figure is estimated to be $886bn with business accounting for $690bn.
The return from using advanced technologies is considerable. Looking at the pharma industry, Accenture estimates that scaled use and redesigned workflows will mean that medicines can be brought to market four years faster, earning an extra $2bn for each successful drug. Costs of $2.6bn to $6.7bn could also be scythed by up to 45 per cent.
With such large sums at stake, companies will have to think carefully about where and how they spend their cash to reach the consumer.
In a previous report we studied the role of technology in marketing and how businesses can use technology tools to foster loyalty with personalised messaging and to create holistic cross-channel strategies.
Turning to the front end of the product cycle, this report will examine how technology can help providers update products more efficiently and successfully at each stage of R&D.
We are all technology companies
Mindset is an important factor in innovation. Sean Ammirati, professor of entrepreneurship at Carnegie Mellon University, Pennsylvania, says an entrepreneurial culture inside the R&D function helps companies to innovate — even the large ones. Teams with such a mindset are more likely to come up with transformational rather than incremental product developments.
Ammirati, who has founded several machine-learning start-ups, says many companies do not make the necessary investment in adjacent and transformational innovation. He cited a 2012 paper by Harvard Business Review, which stated: “Companies that allocated 70 per cent of innovation activity to core initiatives, 20 per cent to adjacent ones and 10 per cent to transformational ones outperformed their peers.”
Technology companies should invest more in these two areas, the same research said. Given today’s ubiquity of technology, Ammirati says that every business should think of itself as a technology company when it decides its R&D budget.
Identify your target
It is important to identify what a product is trying to achieve and who is the target — this might not always be obvious. Pella, the Iowa window and door maker, has designed a mechanism that treats the installer rather than the homeowner as its customer. Based on observations and responses, Pella’s new window is easy to install from the inside rather than the outside of a property, reducing the risk to workers when putting windows into tall buildings.
Targets are important for the R&D process, too. This should include deciding if the objectives include cutting the cost of materials, the cost of engineering, time to market, or all three. Having this in mind helps with setting key performance indicators to assess whether a process works.
The role of data
Customer needs should always be the inspiration for product development. The more data that is available, the easier these are to define. Data is critical to any technology strategy. It can, for instance, alert companies to what customers are seeking and what is in demand.
Online marketplaces have extensive access to data about purchasing information and shopping searches which can give them an advantage over the vendors who use their sites. As we observed in our report on the platform economy, there is value in brands hosting their own websites as a means to retain and interpret customer data. This can give instant insights into modifying or adding to what they offer. For example the bra company Lively introduced strapless bras to its range after it found that many women were searching for these. Tommy John, which makes men’s underwear, found that women liked its products too and it added second-skin “boyshorts”, tees and pants aimed at females.
When collecting or compiling data, it is important for it to be clean. This is especially the case when deploying artificial intelligence systems which are in essence statistical models that feed on data. “This means your team needs to care about data,” Ammirati says. “If your team are being sloppy with how they enter data you’re actually causing downstream problems.”
Increasingly products can be developed for a “segment of one” using customer information. Skincare ranges such as Fenty Beauty offer products to suit a customer’s skin tone using a combination of image recognition and machine learning. Other companies use customer-supplied data or insights gleaned from previous purchases to suggest or customise products. Nike By You allows customers to create their own trainers from a palette of styles, colours and designs.
The co-creation of products goes beyond personalisation, This is where customers advise companies what they want by specifying modifications beyond those given in a drop-down list. Ikea is among the groups that have co-created products with customers for several years.
At a summit on AI hosted by Bloomreach, Azita Martin, vice-president of AI for retail at Nvidia, said the next challenge for many companies would be in fulfilling highly personalised orders — essentially for a market of one — quickly enough to satisfy the consumer. She said the correct forecasting, faster throughput in distribution centres (using computer vision, robotics and simulation) and last-mile delivery were areas where AI could give retailers more agility.
From concept to reality
People can still come up with product ideas independently of data. Technology can help to accelerate these to market and AI is a powerful tool in that process.
While human oversight will remain as important for R&D as it is for the creation of marketing content, generative AI based on large language models will be invaluable as a “conversation starter”. Presented with an idea or even a vague concept, generative AI can help with brainstorming to help develop a product, undertake market research to find if there are similar items, identify and analyse competitors and give thoughts on how to create a difference. AI can provide many versions of a product and suggest modifications to suit a niche that a human designer might not have considered.
Once the product concept has been honed, AI can help to devise market-testing strategies as well as accelerate product testing and design. It can create and test iterations of a product at a speed far faster than a human. It can suggest materials and sourcing as well as manufacturing processes.
This is particularly helpful for start-ups and smaller businesses. The founder of Skittenz, a company that makes mitten covers to enliven ski gloves, used AI to investigate which materials and manufacturing processes might be suitable to bring the product to market.
Supercharging with AI
AI is also invaluable for an established R&D team, even those that deploy more traditional systems and programs, which we will cover below. These are increasingly augmented by AI, enabling designers to devise more options more quickly than the underlying software alone. Ammirati, who has specialised in both corporate innovation and AI in the enterprise, says that in the past three years these two workstreams have merged and can no longer be considered separate areas of expertise.
Based on findings from a forum of R&D leaders in July 2024, McKinsey says that both analytical and generative AI are likely to have a big effect on innovation outcomes. It estimates increased market fit of up to 50 per cent, product performance improvements of 15 to 60 per cent, increased workplace productivity of up to 50 per cent and up to 40 per cent reduction in time to market. Simulations and deep learning surrogates in particular increase testing capabilities. Bain research makes similar findings, with engineering hours reduced by up to a fifth and cost falling by between 5 and 30 per cent.
Both stress that stakeholders’ buy-in is essential in the digitalisation process. This might be more easily achieved in a workforce if leaders are clear that tech, and AI specifically, is a long way from replacing people. Among other reasons, this is due to the continued, if diminished, incidence of hallucinations (when AI creates information and presents it as fact). “Human plus AI” will be the future, especially for R&D.
Ammirati says: “The right mental model is to think of generative AI as a co-founder, or what is often described as ‘human in the loop AI’. So think of it more like a brainstorming buddy, more like someone who automates first drafts than does all the work for you.”
While AI will not take your job, he says, companies using the tool will be “incredibly disruptive” to those who are not. “This is not something that you can only train your executives on. This is something that everybody in the organisation needs to be aware of.” To keep up with the competition, never mind stay ahead, business leaders must educate their workforce in how to use AI.
This does not necessarily mean teaching prompt engineering, which Ammirati believes will become obsolete as interfaces change. Indeed a new interface is already here in the form of AI agents. These LLM-based AI apps can summon the help of tools and data and so are not bound by the constraints of the most recent update. Over time they learn user preferences, retain conversations for context and can access and deploy other programs and information autonomously in response to user requests, optimising workflows and creating subtasks.
Too many businesses are not ready to harness AI effectively. The State of AI 2024, a global survey of 300 senior executives by Searce, the modern technology consultant, found that while all UK and US respondents were using or planning to use AI, only 14 per cent of companies had managed to scale beyond the pilot stage.
Impediments included lack of talent, especially for UK respondents, which suggests those companies that are quick to invest in educating their current workforce will have an advantage. Badly managed data and poorly prioritised uses were further barriers to successful adoption.
Despite the hurdles, adoption is no longer optional. For those that are still not convinced, Ammirati says we have reached a “general technology inflection point”. Think of it as similar to when the internet arrived. “The businesses who said no, we’re just going to avoid that internet thing — most of them, unfortunately, are not around.”
Technologies available for R&D
Steps in the R&D process such as testing and predictive modelling increasingly take advantage of AI, including machine learning and statistical modelling. These are enabled at greater speed and scale by the increased processing power of cloud computing. While this is not a replacement for expert oversight, GenAI provides an intuitive interface for those who may not have the technical training that was once essential even for early product development. The technologies include:
Market Research
Digitally distributed market surveys, such as those offered by SurveyMonkey, Google Forms and Typeform to name just a few, offer huge reach and enhanced analytics.
Design
CAD-CAM software such as that provided by Autodesk, Siemens and Trimble is used for design in fields such as engineering, architecture and manufacturing. Many of the programs incorporate AI which quickly provides a greater number of alternative designs. So-called generative design deploys AI algorithms derived by machine learning, which can come up with designs optimised for different parameters such as material economies or strength. Provided the engineer specifies minimum requirements and constraints such as manufacturing process, loads and flexibility, the system can offer many variations, some of which are likely to be novel.
Prototyping
This too “will now almost always start in the digital environment”, says Mark Ridley, a former chief technology officer of the Financial Times who is now a technology consultant. Pure digital prototyping, he says, can massively reduce cost, which will allow many more attempts. Machine-learning models facilitated by cloud capacity have enabled more digital experimentation in the R&D process. Cloud computing means companies no longer need big budgets to buy in-house servers for complex computations. “The technology of the cloud allowed for really significant innovation . . . because it provided democratised technology, which could then be used by small entrepreneurial teams.” Ridley adds: “The beauty here is that with the increase in computational power [and] the availability of software to do these things, designers can now augment more traditional models like computational fluid dynamics with ML-based generative design.”
Simulations
These are increasingly popular for testing marketing strategies, such as deciding which wording best targets the customer and which communications medium to choose. Simulations use A/B testing (comparing different versions of a strategy). They assume that a product is already viable and that the sales performance will be affected by marketing rather than how the product is designed and performs.
Simulations can also be used for product development, testing for variations in materials and design — often using the same software as that used to design the product. Engineering software from providers such as Ansys and Matlab enables designers to render objects virtually and simulate systems in which to test and analyse aspects of their behaviour in different environments, for instance their fluid and thermal dynamics. The value in such software lies in being able to test without a physical prototype.
Understanding market receptiveness to the product is the next step. While adjacent to marketing, this is less based on testing a strategy and more designed to determine viability. Modelling software can help in areas such as comparing pricing and performance against competitors. It can also examine the effect of different economic environments and competitor actions. It is easier to test material or design behaviour in a simulation than it is to try to assess human behaviour, which is so much more unpredictable.
While simulations can be a processing burden, augmented capacity can come from cloud computing.
3D printing
Once simulations and tests have run successfully there is still more that can be done without necessarily investing in the actual product. Ridley says processes used to require drawings followed by clay modelling. Digitalisation has significantly lowered the barriers to production. “The clay model requires artistry and deep expertise. And what we’re seeing now is that it is easier to buy patterns and cheap technology off the shelf on a credit card. That enables more users and small groups to do the innovation themselves.”
GenAI interfacing with 3D printing might eventually allow a creator to discuss the attributes it wants from a given product, Ridley says. They might for instance ask the computer to come up with variants of a mop that simplify aspects such as cleaning into corners, rinsing or applying detergent.
To produce injection-moulded plastics components, normally requiring expensive and specialised machinery, Bosch has developed advanced 3D printing with AI algorithms. These can adjust substrate inputs, heat and pressure in real time, ensuring that prototype quality is as good as the end product. This also allows for small batch production before investment in large-scale facilities. Separately Bosch has developed ceramic 3D printing which models the different shrinkages experienced when an object is kiln-fired.
Digital twinning
A digital twin is a virtual model of a planned or actual real world product or process. It is a step up from a straight digital simulation as it can optimise integration, testing, monitoring and maintenance of facilities such as supply chains or power plants. A twin is particularly useful in working out lifecycle management, modelling in theory how a product might behave over time, updating itself as necessary. The twin emulates, replicates, observes and evaluates a product or process and highlights opportunities for change.
Demand is growing. Fortune Business Insights estimates that digital twinning will propel an 82 per cent increase in the market for computer-assisted design and product lifecycle management software, helping it to reach $26.4bn by 2030. North America is expected to account for one-third of the overall market.
While still early days, EY highlights the industrial metaverse as the next phase in digital twin technology, with an asset rendered digitally in virtual space. Siemens, a provider of digital twin technology, partnered in 2022 with Nvidia to create an industrial metaverse, allowing users to see their creations in an immersive digital universe.
Collaboration software
Communication and collaboration across an organisation between members of different units is far easier with technology. At the most basic level, Slack allows for discussion between colleagues. There are several more specialised programmes for innovation and idea management, such as Miro, Braineet and Ideanote. It is worth noting that while these programs help humans to share knowledge and advance ideas, they do not generate or develop concepts themselves.
This type of knowledge banking is still helpful if it allows companies to find historical data easily. “The key to innovation is not success,” says Ridley.
“The key is that you fail very quickly and you learn from every one of those experiments. You want to keep every failed experiment — because the failed experiments are sources of learning.”
It also helps to keep a record of how and why changes were made to products to avoid spurious reversals — and to be sure that the data is accurate. Finally, AI can help with checking that existing patents or regulations are not being infringed.
What’s next?
Even as AI makes strides in boosting capacity to create more products at higher speed, quantum technologies could add new dimensions. The UK has invested in this area for a decade and in July the government announced funding of £100mn to establish quantum hubs to push forward development.
We are still some way from understanding the full potential of quantum computing but it could help with material and drug discoveries, modelling existing materials and chemical processes.
Quantum sensors, which can be far more sensitive than regular sensors, are already used in some commercial applications. These include brain-scanning, where quantum sensors allow for a wider range of diagnostic environments, and gravity sensing, which can be used to scan for subsurface composition, for instance in the construction industry. Electric battery researchers have already deployed quantum sensors to analyse microcurrents and improve production yields.
There are some obstructions to wider-scale application, including the hypersensitivity of quantum sensors, which makes them difficult to use in some environments, their demand for power and their current complexity. Nevertheless we could see developments that allow for more widespread commercial usage within two to five years, depending on the application.